HIMA Workshop 2021
HIMA WORKSHOP - How to collaborate with image analysis scientists?
Course Schedule
Date |
Time |
Topic |
Presenter
|
12:00pm |
Introduction |
Metin Gurcan, PhD |
12:05 |
Pathologist's Perspective |
Michael Feldman, MD, PhD |
12:15 |
Why and what can we do? |
Scott Doyle, PhD |
12:45 |
Evaluation of medical image analysis systems |
Berkman Sahiner, PhD |
13:15 |
How to read a machine learning paper? |
Andrew Janowczyk, Phd |
13:45 |
15-minute break |
|
14:00 |
How can we do it? - Part 1 |
Khalid Niazi, Phd |
14:30 |
How can we do it? - Part 2 |
Spyridon Bakas, PhD |
15:00 |
End |
|
Target Audience
Pathologists, House Officers, Medical Students, other healthcare professional
Introduction
Metin Gurcan, PhD
Wake Forest School of Medicine
This course is designed for pathologists and pathology trainees who want to collaborate with image analysis scientists successfully in developing machine learning and artificial intelligence techniques and learn how to use them in their practice effectively. After hearing the perspective of a pathologist who has been collaborating with image analysis scientists for over 15 years, the participants will learn about the core terminology, basic techniques and evaluation methods of image analysis. Finally, there will be a lecture on how to critically review the literature in this field.
After completing this activity, participants will be able to apply new methods for confidently collaborating with image analysis scientists, utilize evaluation methods when reading a machine learning paper, and use cutting-edge machine learning and AI techniques effectively in their practice.
Objectives:
- Learn how to collaborate with image analysis scientists successfully
- Describe the benefits of computational pathology
- Define the core terminology of computational pathology
- Compare AI vs. ML vs. DL vs. Statistics vs. Pattern Recognition
- Learn how to read a machine learning paper
Pathologist‘s Perspective
Michael Feldman, MD, PhD
University of Pennsylvania
This introductory talk will provide the perspective of a pathologist who has been collaborating with image analysis scientists for over 15 years.
a) How do pathologists partner with imaging scientists
b) Separating the wheat from the chaff, how do I think about "use cases" selection
c) Getting out of your comfort zone
Why and what can we do
Scott Doyle, PhD
University at Buffalo SUNY
When starting a computational pathology project, the goals of the project must be clearly and precisely defined. This talk will introduce the benefits of computational pathology, define the core terminology you will hear about for the rest of the course, and use real-world examples to demonstrate how these systems can help advance pathology and improve patient care. It will also touch upon the perennial question: Will an AI replace traditional pathology?
1) Why are we interested in computational pathology?
a) Improve efficiency, reduce clinician burnout, distribute the workload
b) Computational pathology is part of the atoms to anatomy view of medicine
i) Smaller-scale: mapping to genomics
ii) Higher scale: mapping to Anatomy (computational radiology/oncology)
c) Pathway to Integrated Diagnostics and virtual tumor boards (connection/comparison with various clinical/diagnostic tests)
2) What does one need to know before starting a computational pathology project?
a) Terminology and Project Goals
i) Localization, Detection, Segmentation, Classification, Regression
ii) Defining endpoints/labels for each of the above
iii) Understanding data: Scope, scale, size
b) What is the contribution of early-stage clinicians in an image analysis project?
i) What prerequisites are there?
3) AI vs. Humans: The ten-year horizon for computational pathology
a) AI is meant to complement traditional pathology while it learns
b) Medical responsibility lies with the pathologist
c) Interpretability is key for usage and reliability
d) Beyond ten years, will pathology be the same?
Evaluation of medical image analysis systems
Berkman Sahiner, PhD
US FDA
Proper evaluation of computerized medical image analysis systems helps users understand the system performance and provides feedback to the designers for potential improvement. This talk will cover some of the fundamental components in evaluating computerized medical image analysis systems, including data sets, reference standards, task-based figures of merit, and statistical considerations.
1) Data set representativeness and hygiene
a) Representativeness with respect to what is claimed
b) Training, tuning, and testing
c) Independence between training and test
2) Reference standard
a) Disease status
b) Location and extent of regions/structures of interest
c) Combining multiple clinicians‘ interpretations for ground truth
3) Figures of merit
a) Task dependence
b) Figures of merit for binary classification
c) Figures of merit for image segmentation
4) Statistical considerations
a) Pre-defined statistical analysis plans
b) Reporting confidence intervals
How to read a machine learning paper
Andrew Janowczyk, PhD
Case Western Reserve University
This talk will discuss concepts associated with how to read a machine learning paper to understand better and assign an associated level of confidence to the approach. The lecture will follow concepts from the JAMA paper "How to Read Articles That Use Machine Learning: Users‘ Guides to the Medical Literature" (https://jamanetwork.com/journals/jama/article-abstract/2754798).
1) Are the results presented in this study valid?
a) Appropriate reference standard?
b) Spectrum of patients included in the study?
c) Completely independent validation set?
d) Complete reporting of results (likelihood ratios, sensitivity, specificity for the test results, etc.)
e) Will the reproducibility of the test result and its interpretation be satisfactory in my setting? Reliability and feature stability
2) Validation approaches
a) Hold-out
b) Cross-validation
c) Training vs. tuning vs. validation
3) Possibility of bias?
a) Batch effects
b) Blind comparison with a reference standard
c) Did treatment standards change over time?
d) Appropriate spectrum of patients?
e) Were the methods for performing the test described in sufficient detail to permit replication?
How can we do it?
Part I:
Khalid Niazi, PhD
Wake Forest School of Medicine
The lecture will cover how to interact with image analysis scientists. I will go into various aspects of data preparation and processing. We will also discuss filtering, feature extraction, and their connections to deep learning.
1) Structuring/Positioning AI vs. ML vs. DL vs. statistics vs. Pattern Recognition
2) Data
a) Quality: Garbage in - garbage out
b) Size: sufficient set
c) Easy goes first
d) Sources of staining variation
e) Normalization
3) Image Content
a) Filters
b) Feature Extraction
Part II:
Spyridon Bakas, PhD
University of Pennsylvania
The first part of this session will focus on collaborative machine learning as a potential paradigm shift in multi-institutional computational collaborations, where the data of each collaborating institution are always retained within the institution. Then the second part will attempt to offer an overview of publicly available software packages aiming at expediting computational analyses.
1) Multi-Institutional Collaborations
a) Why are they essential?
b) Current paradigm: Bringing data to the algorithms
c) Federated paradigm: Bringing algorithms to the data
2) Tools Facilitating Computational Pathology Analyses
a) Open-source vs. Close-source
b) F.A.I.R. criteria
c) Tool examples
d) Plug to ITCR as an NIH program
HIMA Presenters
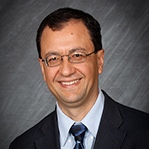 |
Metin N. Gurcan, PhD Director, Center for Biomedical Informatics Professor, Department of Internal Medicine, Biomedical Engineering, Pathology Wake Forest School of Medicine
|
Dr. Metin Gurcan is the founding Director of the Center for Biomedical Informatics and Professor of Internal Medicine, Pathology, and Biomedical Engineering at Wake Forest School of Medicine and Director of the Clinical Image Analysis Lab (http://tsi.wakehealth.edu/CIALab/). Previously, he was Professor of Biomedical Informatics and Pathology, Director of Division of Clinical and Translational Informatics at The Ohio State University. Dr. Gurcan is an internationally recognized researcher and educator in the fields of medical image analysis, artificial intelligence, and biomedical informatics. His research has been supported by NIH NCATS, NCI, NIDCD, NHLBI, NBIB, NIAID, DOD, as well as awards from several nonprofit organizations. He is the author of over 200 peer-reviewed publications, book chapters and was awarded three patents for his inventions in medical image analysis. Dr. Gurcan received his BSc. and Ph.D. degrees in Electrical and Electronics Engineering from Bilkent University, Turkey, and his MSc. Degree in Digital Systems Engineering from the University of Manchester Institute of Science and Technology, England.
Dr. Gurcan is the recipient of several awards, including the British Foreign and Commonwealth Organization Award, NCI caBIG Embodying the Vision Award, NIH Exceptional, Unconventional Research Enabling Knowledge Acceleration (EUREKA) Award, Children‘s Neuroblastoma Cancer Foundation Young Investigator Award, The OSU Cancer Center REAP Award, and Pelotonia Idea Award. He is a Fellow of SPIE and a senior member of IEEE and AMIA. He currently serves on the editorial boards of Journal of Pathology Informatics and Journal of Medical Imaging, organizes the Pathology Informatics Histopathological Image Analysis (HIMA) workshop, and co-chairs the most largest technical medical imaging symposium, SPIE Medical Imaging.
|
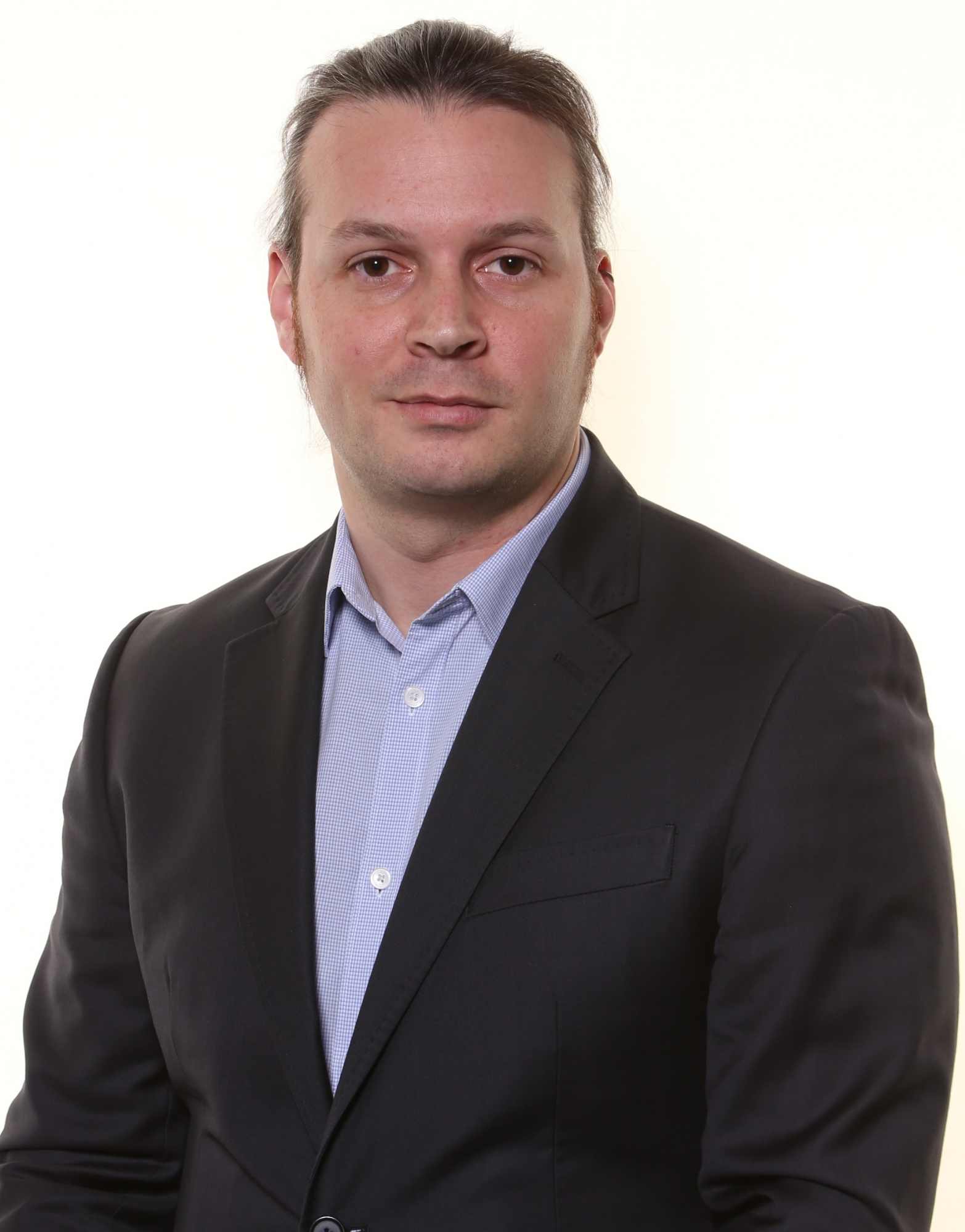 |
Spyridon Bakas, PhD Instructor (Lecturer) at the Center for Biomedical Image Computing and Analytics (CBICA) Perelman School of Medicine of the University of Pennsylvania |
Dr. Spyridon Bakas is currently an Instructor (Lecturer) at the Center for Biomedical Image Computing and Analytics (CBICA), with joint appointments between the Dept. of Radiology and the Dept. of Pathology & Laboratory Medicine at the Perelman School of Medicine of the University of Pennsylvania. He received his B.Sc. degree in Computer Science with highest honors from Kingston University, London, in 2006, his M.Sc. degree in Vision, Imaging and Virtual Environments from University College London, in 2007, and his Ph.D. in Medical Image Computing and Analysis under a collaboration between King‘s College London and Kingston University London in 2014. Since then, he is with the CBICA at UPenn and his research interests focus on the development and application of advanced computational algorithms in oncological imaging, with the intention of improving the assessment, quantification and diagnosis of cancer in the current clinical practice. His work so far has spanned across the areas of image segmentation, feature extraction, statistical analysis, motion analysis, and machine learning techniques applied in brain magnetic resonance (MR), liver contrast-enhanced ultrasound (CEUS), and digitized histopathology, imaging data. The ultimate aim of his research is to contribute towards making diagnostic and treatment decisions more promptly, objectively, and precisely.
Dr. Bakas has received grant funding from the National Cancer Institute of the National Institutes of Health, the National Science Foundation, the Abramson Cancer Center, and the Translational Biomedical Imaging Center of the Institute for Translational Medicine and Therapeutics of UPenn. He has co-authored more than 50 peer-reviewed manuscripts and 40 medical conference abstracts, with more than 450 co-authors from multiple disciplines, institutions, and career stages. He is on the MICCAI Society Challenge Working Group (2020-2021), and on the MICCAI Organizing Committee (2018, 2020-2021), and has been further involved in the organization of various tutorials, workshops, and computational challenges at the Annual MICCAI, ISBI, RSNA, and SNO meetings.
|
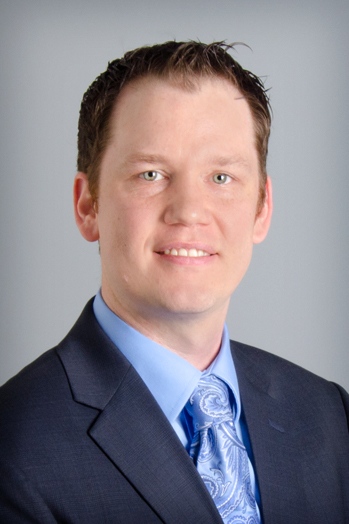 |
Scott Doyle, PhD Assistant Professor, Department of Pathology and Anatomical Sciences University at Buffalo, SUNY |
Scott Doyle received his PhD from Rutgers University in 2010 working on the automated detection and grading of prostate cancer tumor biopsies. Prior to the emergence of deep learning, this work consisted of texture and architectural feature extraction to distinguish levels of differentiation in H&E-stained biopsy images.
The Doyle Lab focuses on quantification of medical imaging, including radiology and computational pathology, with a focus on modeling biological structure. Our goal is to improve image information content for medical applications to drive research, education, and clinical practice with an approach grounded in medical expertise, fusing engineering tools with clinical knowledge to define the problem, develop a solution, and analyze its impact on decision-making. This approach has been successful in bringing together scientists from biological and engineering backgrounds, as well as in international multicenter collaboration to create highly annotated databases of digital pathology images. The Doyle Lab has trained students in multiple fields, from biology and anatomy to math and programming. This ensures that we focus our energy on impactful projects that will improve the level of care provided to patients.
|
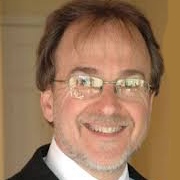 |
Michael Feldman, MD, PhD Professor, Pathology & Laboratory Medicine Vice Chair of Clinical Services Medical Director Pathology Informatics Director Tumor Tissue Bank |
My professional interests revolve around the development, integration and adoption of information technologies in the discipline of Pathology. One of my main areas of interest within this broad discipline has been in the field of digital imaging. We have been studying pathology imaging on several fronts including interactions between pathology/radiology (Radiopathogenomics of prostate cancer and breast carcinoma), development and utilization of computer assisted diagnostic algorithms for machine vision in prostate and breast cancer. More recently we have been developing deep learning methods for complex interrogation of pathology slides both within the cancer domain as well as in cardiovascular and renal pathology. We have also been developing methods to apply multispectral imaging for the analysis of multiplexed immunohistochemistry and immunoflourescence to tissues along with the development of a quantitative system for scoring and analyzing these studies at a cytometric level on surgical pathology slides. The efforts have been recognized by the national funding agencies of the NIHand DOD as well as industry sponsored projects.
|
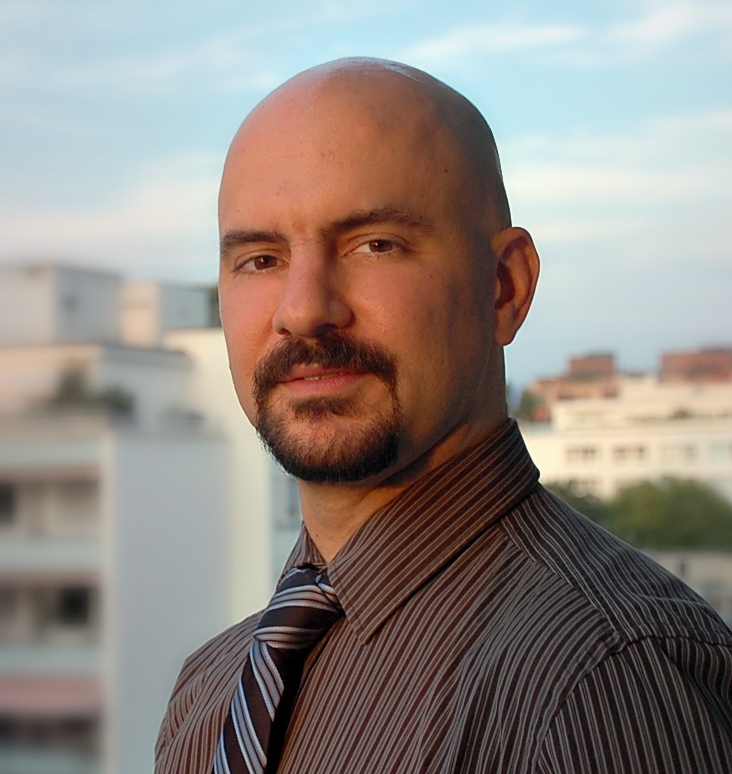 |
Andrew Janowczyk, PhD Assistant Research Professor Department of Biomedical Engineering Case School of Engineering Member, Cancer Imaging Program, Case Comprehensive Cancer Center |
Dr Andrew Janowczyk is an Assistant Research Professor at The Center of Computational Imaging and Personalized Diagnostics (CCIPD) at Case Western Reserve University, and a Senior Research Scientist in the Precision Oncology Center at the Lausanne University Hospital (CHUV), Switzerland. For over 10 years he has applied computer vision algorithms to digital pathology images. One of his areas of expertise is in leveraging deep learning to build computational models to aid pathologists in many common tasks, such as disease detection and grading. Dr Janowczyk‘s 2016 Journal of Pathology Informatics Paper entitled Deep learning for digital pathology image analysis: A comprehensive tutorial with selected use cases (over 30k views), laid out a generalized approach via open-sourced code and datasets to facilitate the development of the next generation of data scientists. In 2018, his tool HistoQC: A quality control pipeline for digital pathology slides received the Innovation Award at the European Congress of Digital Pathology (ECDP). His newer research focuses on the tasks of predicting prognosis and therapy response. He maintains a research-oriented blog, andrewjanowczyk.com, which aims to provide digital pathology related insights, code, and datasets to the research community.
|
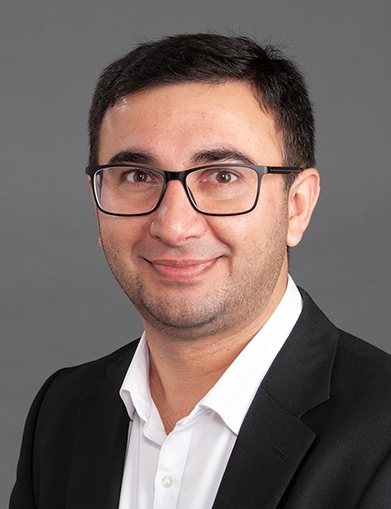 |
Khalid Niazi, PhD Assistant Professor, General Internal Medicine Center for Biomedical Informaticsl |
Dr. Niazi is an Assistant Professor of Biomedical Informatics and Internal Medicine at Wake Forest School of Medicine. His interest lies in developing image analysis and deep learning methods for digital and computational pathology. Unlike conventional computational pathology methods that rely on the detailed annotations of histopathology images, he is developing methods that require little to no annotations.
|
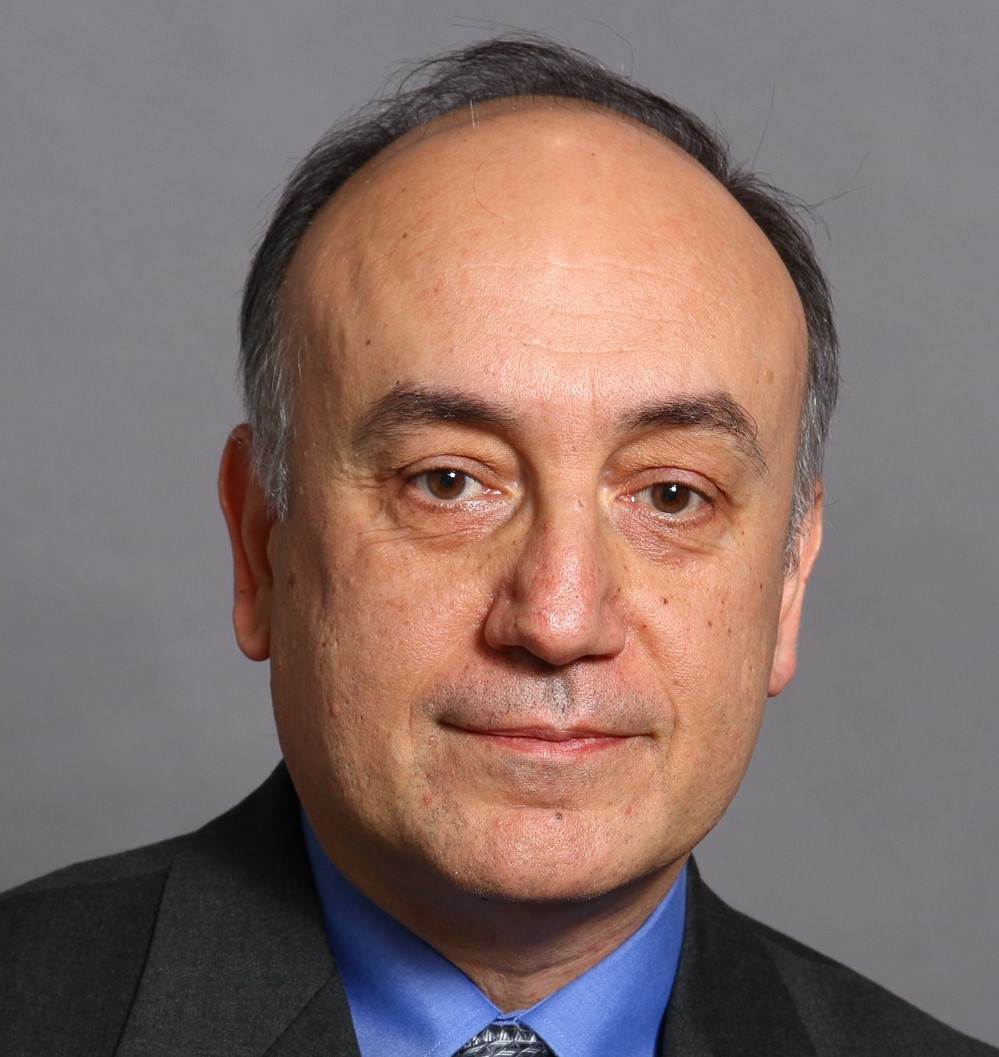 |
Berkman Sahiner, PhD Senior Biomedical Research Scientist U.S. Food and Drug Administration FDA Division of Imaging and Applied Mathematics |
Berkman Sahiner is an senior biomedical research scientist with the Office of Science and Engineering Laboratories (OSEL) at CDRH. He has a PhD in electrical engineering and computer science from the University of Michigan, Ann Arbor. Before joining FDA, he was an Associate Professor with the Department of Radiology at the University of Michigan.
At the Division of Imaging, Diagnostics and Software Reliability at CDRH/OSEL, he performs research related to the evaluation of medical imaging and computer-assisted diagnosis devices, including devices that incorporate machine learning and artificial intelligence. He has authored/co-authored over 130 peer-reviewed journal publications, and is a fellow of the Society of Photo-optical Instrumentation Engineers (SPIE) and American Institute for Medical and Biological Engineering (AIMBE). He has led many projects as the PI of multiple grants, including grants from the NIH, U.S. Army Medical Research and Materiel Command, Office of Women’s Health at the FDA and the Critical Path at the FDA. He is an editorial board member of Medical Physics Journal and a co-chair of American Association of Physicists in Medicine (AAPM) Machine Learning Sub-Committee.
His interests include machine learning, computer-aided diagnosis, image perception, clinical study design, and performance assessment methodologies. |
|